As a cybersecurity professional, you understand the critical importance of real-time threat detection and response in today’s complex digital landscape. Malicious actors are constantly innovating new methods of attack, exploiting the slightest vulnerability in systems and networks. To effectively protect your organization’s sensitive data and infrastructure, you must employ solutions capable of identifying and neutralizing threats the moment they emerge.
In this article, we will explore the cutting-edge real-time threat detection systems being implemented by leaders in the field such as Palo Alto Networks and Check Point. Utilizing sophisticated artificial intelligence, these platforms provide 24/7 monitoring and instantaneous threat neutralization. We will analyze the capabilities of these systems to block exploits, malware, unauthorized access, and more in real time. You will gain key insights into how real-time threat detection can fortify your defenses and allow your team to respond immediately, limiting potential damage and disruption.
The Need for Real-Time Threat Detection Systems
As cyberattacks become increasingly sophisticated, the window of opportunity to detect and mitigate them is narrowing. Real-time threat detection systems that can identify anomalies instantaneously are critical.
Real-time systems can analyze huge volumes of data and events to spot threats as they happen. They use machine learning and behavioral analytics to establish a baseline of normal network activity and user behavior. Anything deviating from the norm can then be flagged as a potential threat. The faster threats are detected, the less damage they can cause.
Accuracy
- Real-time systems are adept at detecting both known and unknown threats. They can identify malicious code and activity signatures that match known threats. They can also detect anomalous behavior that may indicate a zero-day attack. Their self-learning algorithms get better over time at discerning between benign and malicious anomalies. This helps to minimize false positives and ensure security teams focus on actual threats.
Automated Response
- The most advanced real-time systems can take automated actions to contain threats as soon as they are detected. They can quarantine infected endpoints, block malicious IP addresses or user accounts, and patch vulnerabilities. This automated response can help to limit the impact of attacks and buy security teams time to fully remediate the threat.
With cybercrime damages projected to cost $6 trillion annually by 2021, real-time threat detection and response has become essential. AI and machine learning have made these systems highly accurate and scalable, enabling organizations to gain the visibility and control needed to combat today’s stealthy and targeted cyberattacks. Real-time systems represent a vital layer of defense in modern cybersecurity strategies.
1. How Real-Time Threat Detection Systems Work
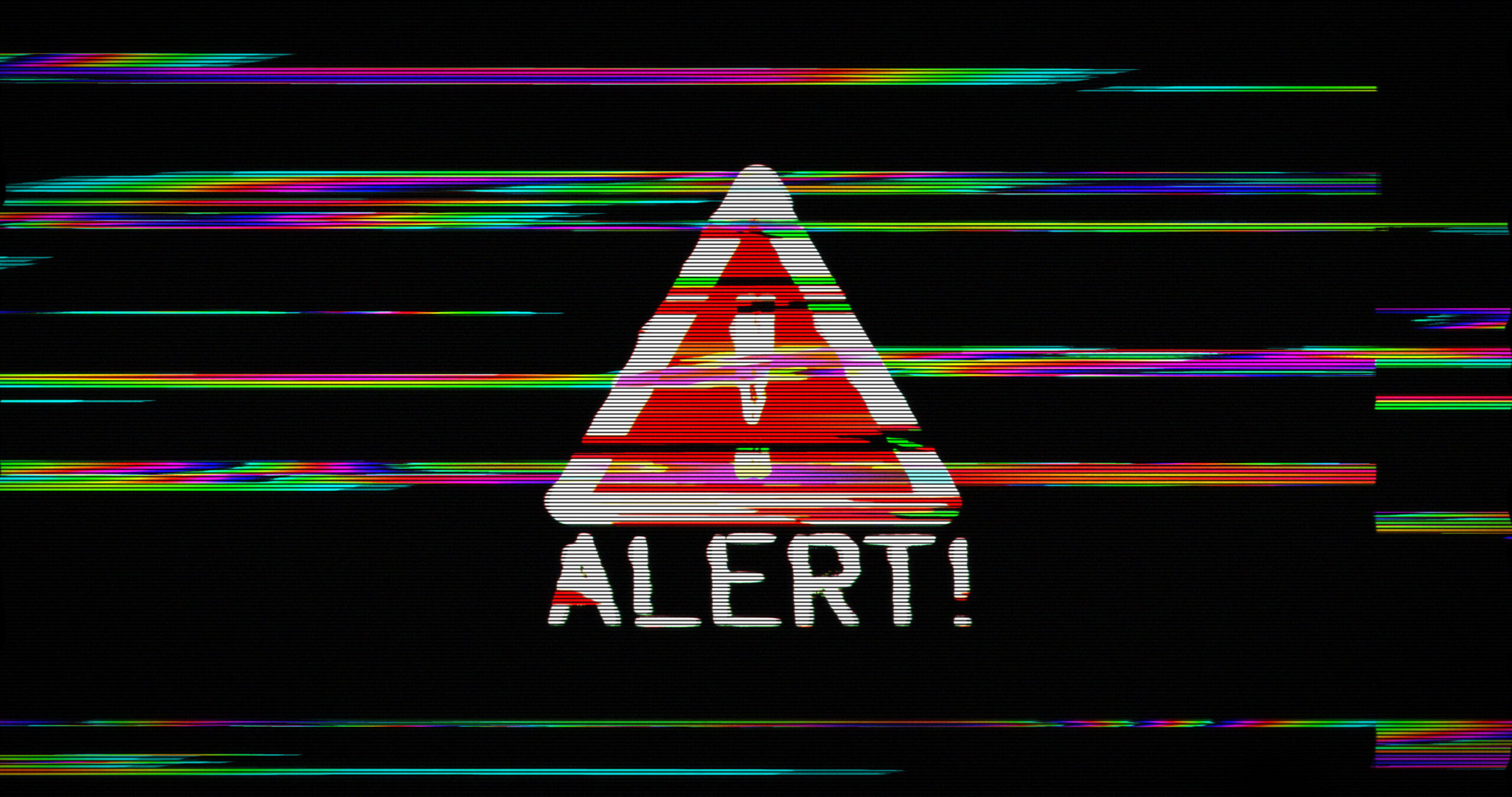
- Real-time threat detection systems utilize advanced AI and machine learning algorithms to identify and mitigate cyber threats instantly. They are able to detect known threats, as well as zero-day threats, by analyzing massive amounts of data in real time and identifying anomalies.
- These systems collect an enormous amount of data from various sources, including network traffic, user behavior, and endpoint activity. The data is analyzed using machine learning models that establish a baseline of normal network behavior and can detect deviations that may indicate threats.
- By analyzing such a large amount of data, the systems can identify known threats like malware, phishing attempts, and DDoS attacks by matching current activity with known threat signatures. They are also able to detect unknown zero-day threats by identifying activity that deviates from the established baseline.
- Once a threat is detected, the system can automatically take action to contain and mitigate it. This may include blocking suspicious network traffic, disabling compromised user accounts, isolating infected endpoints, and more. Security teams are alerted immediately so they can further investigate and remediate the threat.
Continuous Monitoring and Improvement
- Real-time threat detection systems continuously monitor environments and improve over time. As they analyze more data, the machine learning models become more accurate at establishing normal baselines and detecting anomalies. Security teams also provide feedback by validating alerts, which further refine the system. This constant monitoring and model refinement is key to providing robust, real-time threat detection and response.
With the increasing sophistication of cyber threats, real-time threat detection systems have become essential for protecting networks and sensitive data. By utilizing advanced AI and automation, these systems are able to identify and mitigate threats instantly, helping organizations stay ahead of cybercriminals and avoid damage and disruption.
2. Key Features of Real-Time Threat Detection Systems
Continuous Monitoring
- Real-time threat detection systems continuously monitor networks to identify anomalous behavior that could indicate a cyberattack. They utilize machine learning algorithms that establish a baseline of normal network activity and can then detect deviations from that norm. Any suspicious activity is flagged immediately, allowing security teams to take prompt action.
Fast Threat Identification
- These systems are able to detect threats at machine speed, analyzing huge volumes of data to spot threats instantaneously. As soon as a potential threat is identified, an alert is triggered so that security teams can begin investigating right away. The faster threats are detected, the less damage they can inflict.
Automated Threat Prevention
- Some systems are able to automatically block suspected threats to prevent damage, and then alert security teams. They can isolate infected devices, block malicious IP addresses or URLs, and quarantine suspicious files. Automated prevention measures allow organizations to avoid disruption and downtime.
Continuous Tuning
- Real-time threat detection systems continuously learn and improve their detection accuracy over time. As they log more data about network activity and the threats that surface, their machine-learning models become more finely tuned. They get better at distinguishing normal from anomalous behavior and identifying new threat patterns. Continuous tuning allows these systems to keep up with the rapidly evolving threat landscape.
Integrated Solutions
- The most robust real-time threat detection systems provide integrated solutions with features like a centralized management console, detailed reporting and analytics, threat intelligence feeds, and tools for incident response. Integrated solutions give security teams a comprehensive set of capabilities for detecting and mitigating threats across the entire organization.
In summary, real-time threat detection systems offer continuous monitoring, fast threat identification, automated prevention, continuous tuning, and integrated solutions. By leveraging AI and machine learning, these systems can spot threats instantly and help organizations avoid disruption from cyberattacks. With technology advancing rapidly, real-time threat detection has become essential for cybersecurity.
3. Real-world examples and Case Studies
I. Palo Alto Networks
- Palo Alto Networks is a leader in real-time threat detection. Their systems utilize machine learning and behavioral analytics to detect anomalies and block cyber threats. One example is their DNS Security solution, which leverages AI to detect malicious domains and block access. It identifies suspicious domains by analyzing features like domain age, request frequency, and registration details. Once detected, the solution automatically blocks access to prevent data exfiltration or malware delivery.
II. Check Point
- Check Point is another firm that provides real-time threat prevention solutions. Their Anti-Bot blade uses AI to detect botnet activity and block automated threats like credential stuffing instantly. It analyses traffic patterns to identify bot-like behavior and then blocks the source IP address. Check Point has reported that its solution has blocked over 70 million botnet-related cyber threats to date, highlighting the prevalence of these attacks.
III. CrowdStrike
- CrowdStrike Falcon Prevent Next-Gen AV solution utilizes AI and indicators of attack to detect and block threats in real-time. It analyses executable files, scripts, and malicious documents using AI to determine malicious intent before the file is opened or the code is executed. One case study found that Falcon Prevent caught 83% of malware that traditional antivirus solutions missed over a 4-week period. Their solution was also able to block 92% of malware within 60 seconds of delivery.
In summary, real-time threat detection solutions that harness AI and behavioral analytics are enabling companies to identify and neutralize cyber threats faster than ever before. The examples provided by Palo Alto Networks, Check Point, and CrowdStrike demonstrate how these systems can block threats like malicious domains, botnets, malware, and more—all in real-time. By stopping threats before data can be accessed or stolen, these solutions help minimize the impact and remediation costs of cyber attacks. Overall, real-time threat detection powered by AI is transforming cybersecurity.
4. Choosing the Right Real-Time Threat Detection System for Your Organisation
When implementing a real-time threat detection system, selecting a solution that aligns with your organizational needs and cybersecurity maturity is crucial. Assess your current cybersecurity capabilities and any regulatory compliance requirements to determine what features and functionality are most critical.
Evaluate Your Threat Landscape
- Conduct a risk assessment to gain visibility into your organization’s threat landscape and potential vulnerabilities. Analyse historical data on the types of threats you have faced, as well as current weak points in your infrastructure. Solutions with robust threat intelligence and behavioral analysis capabilities may be ideal if you face a high volume of unknown threats. For organizations with more basic needs, a solution focused on signature-based threat detection may suffice.
Consider Deployment Options
- Real-time threat detection systems are available as on-premises solutions, cloud-based services, or hybrid deployments. On-premises solutions may appeal to organizations with strict data security and compliance needs. Cloud-based services reduce infrastructure costs but may present data privacy concerns. A hybrid approach utilizes both on-premises and cloud-based components, balancing flexibility, cost efficiency, and control.
Determine Required Features
- Once you understand your key priorities, evaluate potential solutions based on must-have features. This may include threat intelligence feeds, sandboxing, endpoint detection and response, user and entity behavior analytics, and incident response automation. Additional functionalities like network traffic analysis, data loss prevention, and deception technology provide extra layers of protection.
Plan for Effective Implementation
- The success of your real-time threat detection system relies on proper deployment and configuration. Carefully consider how the solution will integrate into your infrastructure and any changes required to policies, procedures, and daily operations. Provide comprehensive training to security teams and allocate resources to monitor, manage, and optimize the system over time. With the right solution and planning in place, real-time threat detection can significantly strengthen your cyber defenses.
What’s The Verdict?
So, in summary, real-time threat detection systems powered by artificial intelligence represent the future of cybersecurity. By leveraging machine learning algorithms to instantly identify and respond to the latest malware and hacking techniques, these cutting-edge solutions provide robust protection for businesses and organizations. While AI-driven systems require significant investment, their capability to operate autonomously and make split-second decisions delivers unparalleled safeguarding of critical networks and data. As threats continue to evolve at a rapid pace, partnering with an industry leader in real-time detection will ensure your systems remain resilient and your sensitive information stays secure. With insight into both existing and emergent risks, you can confidently continue innovating without compromise. By implementing these advanced protections now, you guarantee comprehensive coverage both today and into the future.