As an enterprise leader, you find yourself at the helm of a new algorithmic age, where artificial intelligence and machine learning shape decisions and steer strategy. This technological transformation brings promise and peril; while intelligent systems can drive innovation and progress, they pose ethical risks if deployed without care. To chart these turbulent waters, you must balance the siren song of AI’s potential with the harsh realities of its pitfalls. By taking an ethical approach, instituting responsible governance, and opening a dialogue on transparency and accountability, you can harness AI’s power while upholding your values. The path ahead is complex, but with wisdom and foresight, you can progress responsibly into an intelligent future.
The Ethical Dilemmas of AI in Business
Bias and Fairness
- AI systems can reflect and amplify the biases of their human creators. If the teams building AI systems lack diversity or train on skewed data, the systems they develop may discriminate unfairly. Hence, businesses must audit AI systems for unfair bias and make corrections to promote equitable outcomes.
Job Disruption
- As AI systems take over routine tasks, job opportunities will decrease over time. However, AI will also create new jobs, such as AI ethicists and data scientists. Companies have a responsibility to retrain and reskill workers displaced by AI. They should also create new jobs and career paths to employ human talent.
Lack of Transparency
- Many AI techniques are based on complex algorithms and neural networks that are opaque and hard for people to understand. When an AI system makes an important decision, it can be difficult to know why. This lack of explainability poses risks, and companies should make efforts to develop more transparent and interpretable AI systems. Explainable AI is an active area of research that aims to address this issue.
Bias in Data
- AI systems are only as good as the data used to train them. If a company’s data is unrepresentative or skewed, the AI systems built on that data may discriminate unfairly. Companies must ensure that AI training data is fair, accurate, and representative. They should consider approaches like data audits, correcting imbalances, and including more diverse data.
Responsibility and Accountability
- As AI systems become more autonomous and powerful, questions of responsibility and accountability become pressing. If an AI system causes harm, who is responsible? The company that developed the system or the human users? Laws and policies around AI accountability are still evolving. Furthermore, companies building AI systems should proactively address responsibility issues to avoid legal trouble and public backlash. Responsible practices include human oversight of AI systems, monitoring and testing for issues, and correcting problems that arise.
Key Principles for Ethical AI in the Enterprise
1. Transparency and Explainability
In Order to trust and adopt AI, its decisions and predictions must be transparent and explainable. When designing AI algorithms, companies must provide insights into how they function and arrive at outputs. Companies must also explain the key factors that drive their AI systems’ behaviors.
2. Bias and Fairness
AI models should be free from unfair bias and make impartial decisions. If not designed carefully, algorithms can reflect and even amplify the biases of their human creators. Enterprises must evaluate AI systems for unfair impacts on groups of people based on characteristics like race, gender, age, and socioeconomic status. They should then take steps to address those issues.
3. Accountability
With AI making an increasing number of autonomous decisions, accountability is crucial. There must be clarity on who is responsible for AI systems’ behavior and actions. This could be the team that developed the algorithm, the business leaders who implemented it, or the company as a whole. Policies and governance frameworks are necessary to determine accountability.
4. Privacy
The collection and use of data to train AI models raise privacy concerns. So, data must remain confidential and secure and only used for the purposes for which individuals have given consent. To safeguard people’s personal information and comply with laws, it will require strict data governance and oversight like GDPR (General Data Protection Regulation). Anonymization and other privacy-enhancing techniques can help reduce risks.
5. Ethical Outcomes
Ultimately, AI should align with ethical values and prioritize beneficial outcomes for society. Therefore, we musn’t deploy algorithms that potentially cause harm to human well-being.
Companies must consider the societal implications of their AI technologies and make strategic decisions to guide progress in a responsible direction. With the correct principles and governance, AI can be developed and applied ethically.
AI Governance Frameworks: Accountability, Transparency and Fairness
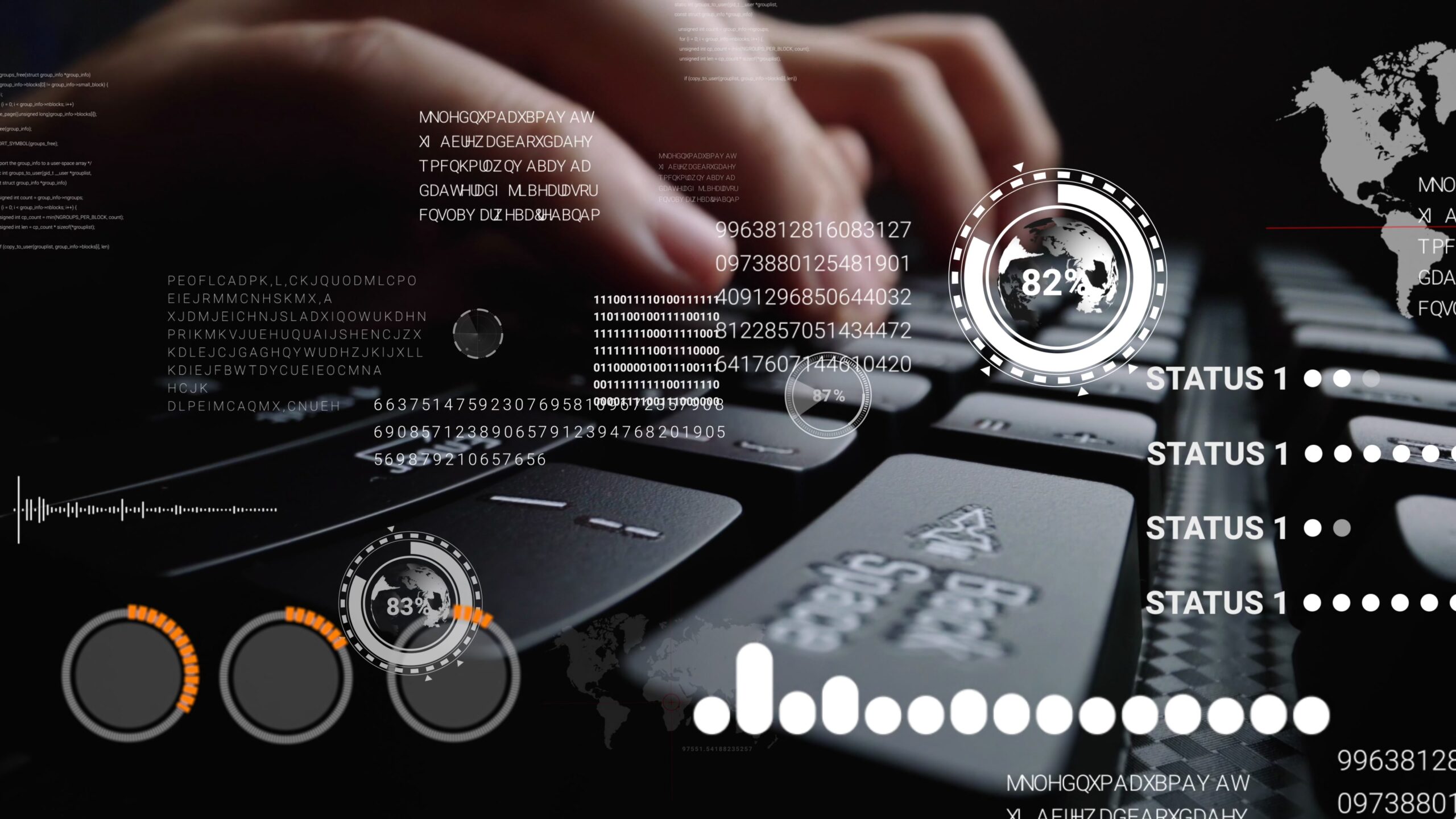
Enterprises must establish AI governance frameworks that consider accountability, transparency, and fairness to ensure AI systems are developed and applied responsibly.
Accountability
- Accountability refers to the policies and procedures in place to determine who is responsible for the outcomes of AI systems. Clearly defined roles and responsibilities are necessary for building, deploying, and monitoring AI systems. This includes data scientists, engineers, legal experts, and executives. Enterprises must also consider accountability to customers, regulators, and the public. Hence why regular audits of AI systems and impact assessments can help determine where accountability lies.
Transparency
- Transparency means that AI systems’ inputs, outputs, and processes can be explained and understood. As such, AI models and the data used to train them should be available for scrutiny. Explanations for predictions and recommendations made by AI systems should be provided. If an AI makes a flawed or biased decision, its reasons must be determinable. Lack of transparency can reduce trust in AI and lead to legal issues. Transparency reports and model documentation help improve clarity.
Fairness
- Fairness refers to the equitable and impartial treatment of individuals by AI systems. Bias in data or algorithms can negatively impact specific groups. That is why AI systems must be developed and tested to ensure fair outcomes across demographic categories like gender, ethnicity, and age. If unfairness is identified, steps must be taken to address it. Considerations of fairness should be embedded throughout the AI development lifecycle. Regular bias audits and inclusive data practices are crucial to achieving fairness.
AI governance frameworks that prioritize accountability, transparency, and fairness help enterprises develop AI responsibly. With the proper oversight and safeguards in place, organizations can navigate the algorithmic age and gain the benefits of AI while minimizing the risks. Overall, governance is about finding the right balance between AI innovation and ethical AI practice.
Mitigating AI Bias: Testing, Auditing and Monitoring
i. Rigorous Testing
- Enterprises must subject algorithms to rigorous testing procedures to mitigate undesirable bias in AI systems. Teams should test AI models with diverse, representative data to identify unwanted correlations before models are deployed. Failing to test with a range of real-world data can allow biases to emerge once the system is in production.
ii. Continuous Auditing
- Testing alone is insufficient. AI systems require ongoing auditing to detect emerging biases. Audits should analyze model predictions, inputs, and outcomes to uncover undesirable patterns. For example, an image classifier could be audited to ensure it does not make consistently inaccurate predictions for specific demographic groups. Audits also provide an opportunity to re-examine the data used to train the model and make improvements.
iii. Monitoring and Feedback Loops
- Responsible AI implementation also demands monitoring systems and feedback loops. Enterprises must track how AI models function in the real world to pinpoint issues and then use feedback to retrain models and fix problems. For example, a recruiting algorithm could be monitored to determine if it systematically disadvantages specific candidates. The insights from monitoring would then inform changes to the model to remove unintended biases before more candidates are affected.
iv. Transparency and Explainability
- Explainable and transparent AI is crucial for identifying and addressing bias. If an algorithm’s predictions cannot be explained, its biases remain hidden. Explainable AI uses techniques like simplifying models to make them more transparent and accessible to audit for prejudices. For enterprise AI, explainability is vital to building trust and accountability.
To steer through the algorithmic age responsibly, enterprises must prioritize AI ethics. Rigorous testing, continuous auditing, monitoring systems, and explainable models are all required to deploy AI that is fair, transparent, and aligned with human values. With issues of bias and unfairness in AI posing real risks, establishing AI governance and oversight has become an urgent need. Enterprises that fail to make ethics fundamental to their AI initiatives will struggle to gain public trust and maintain their social license to operate.
Building a Culture of Responsible AI Innovation
To build AI systems that are ethical and aligned with human values, enterprises must foster a culture where AI ethics is prioritized. This starts at the top, with executives demonstrating a commitment to responsible innovation through policies, governance structures, and oversight.
Leadership Buy-In Executives must understand the risks of irresponsible AI and make ethical AI a strategic priority. They should issue policies on AI ethics, establish cross-functional governance boards to oversee AI projects and provide resources for implementing ethical practices. With leadership supporting responsible AI, teams will have the mandate and means to build trustworthy systems. | Employee Education All employees involved in developing, deploying, or managing AI systems should receive training on ethical principles and practices. Coursework on bias detection, privacy, and transparency helps teams understand why responsible AI matters and how to achieve it. Armed with this knowledge, employees can flag potential issues, suggest solutions, and help audit AI systems. |
Incentivize Ethics Incentives strongly influence behavior and can be used to motivate the consideration of ethics. For example, a portion of executives’ bonuses could depend on responsible innovation metrics. AI teams should also be rewarded for identifying and fixing ethical problems in systems, not just for hitting technical milestones. The overall message must be that AI ethics is valued and impactful. | Continuous Monitoring Responsible AI is an ongoing process that requires continuous monitoring and improvement. Teams should frequently audit AI systems for new ethical risks and issues. They must be willing to halt, fix, or redesign systems that fail to meet ethical standards. Executives and governance boards should review audit findings, track key metrics, and change policies or training programs as necessary to strengthen the culture of ethics. |
With leadership support, education, incentives, and continuous monitoring, enterprises can build a culture where responsible innovation is the norm rather than the exception. AI systems developed in this environment will better align with human values and become more trustworthy.
To Summarize…
As the algorithmic age unfolds, the path ahead for enterprises is clear: embrace AI with open eyes. Navigate new waters guided by moral compasses that point true north to ethical AI. Only with care and conscience can companies fully realize AI’s potential, maximizing benefits to humanity while minimizing unintended harms. The challenges are real, but so are the rewards for those enterprises who confront AI’s ethical frontiers. The future remains unwritten; steer it responsibly. With vision and values, tomorrow’s AI enterprises can uplift society. The time to chart that course is now.
More Stories
Alibaba’s Taobao Surges in Popularity in US as Trump Tariffs Boost Chinese Shopping Apps
As you navigate the ever-changing landscape of global commerce, a surprising trend has emerged in the wake of recent U.S. tariff increases on Chinese goods. Contrary to expectations, these measures have not deterred American consumers from purchasing Chinese products.
Alibaba’s AI Speeds Up Pancreatic Cancer Detection with FDA Breakthrough Status
As you explore the evolving world of healthcare innovation, a major breakthrough in cancer detection now captures global attention. Notably, Alibaba’s research division, Damo Academy, reached a milestone with its AI tool, Damo Panda.
ChatGPT’s Geo-Guessing Surge Sparks Privacy Alarms
As you navigate the ever-evolving landscape of artificial intelligence, a new development in ChatGPT’s Geo-Guessing Surge has emerged, captivating users while simultaneously raising red flags among privacy advocates.
GXS Bank Strengthens SME Offerings with Validus Capital Acquisition
GXS Bank has acquired Validus Capital. This strategic deal is backed by tech giants Grab and Singtel. As a result, GXS Bank now stands at the forefront of SME financing.
Llama 4 Unleashed: Databricks and Meta Forge the Future of Enterprise AI
Databricks’ integration of Meta’s Llama 4 into its Data Intelligence Platform heralds a new era of AI capabilities for organizations worldwide.
Alibaba Accelerates AI Integration in Automotive Industry with Nio and BMW Partnerships
As you navigate the rapidly evolving landscape of automotive technology, you’ll find Alibaba Group at the forefront of artificial intelligence integration.