As you navigate the rapidly evolving landscape of artificial intelligence and cloud computing, a groundbreaking collaboration between tech giants Google, ByteDance, and Red Hat is set to redefine how you deploy and scale AI models. This partnership has yielded significant enhancements to Kubernetes, the industry-standard container orchestration platform, specifically tailored for generative AI workloads. One of the most impactful innovations is the Gateway API Inference Extension, which optimizes model inference through intelligent routing for fine-tuned models. By introducing features like this and improving scalability, these advancements promise to revolutionize your approach to large-scale AI applications. In this article, we’ll explore the key developments that are positioning Kubernetes as the go-to platform for cutting-edge AI deployment and how these changes can benefit your organization’s AI initiatives.
Enhancing Kubernetes for Generative AI Workloads
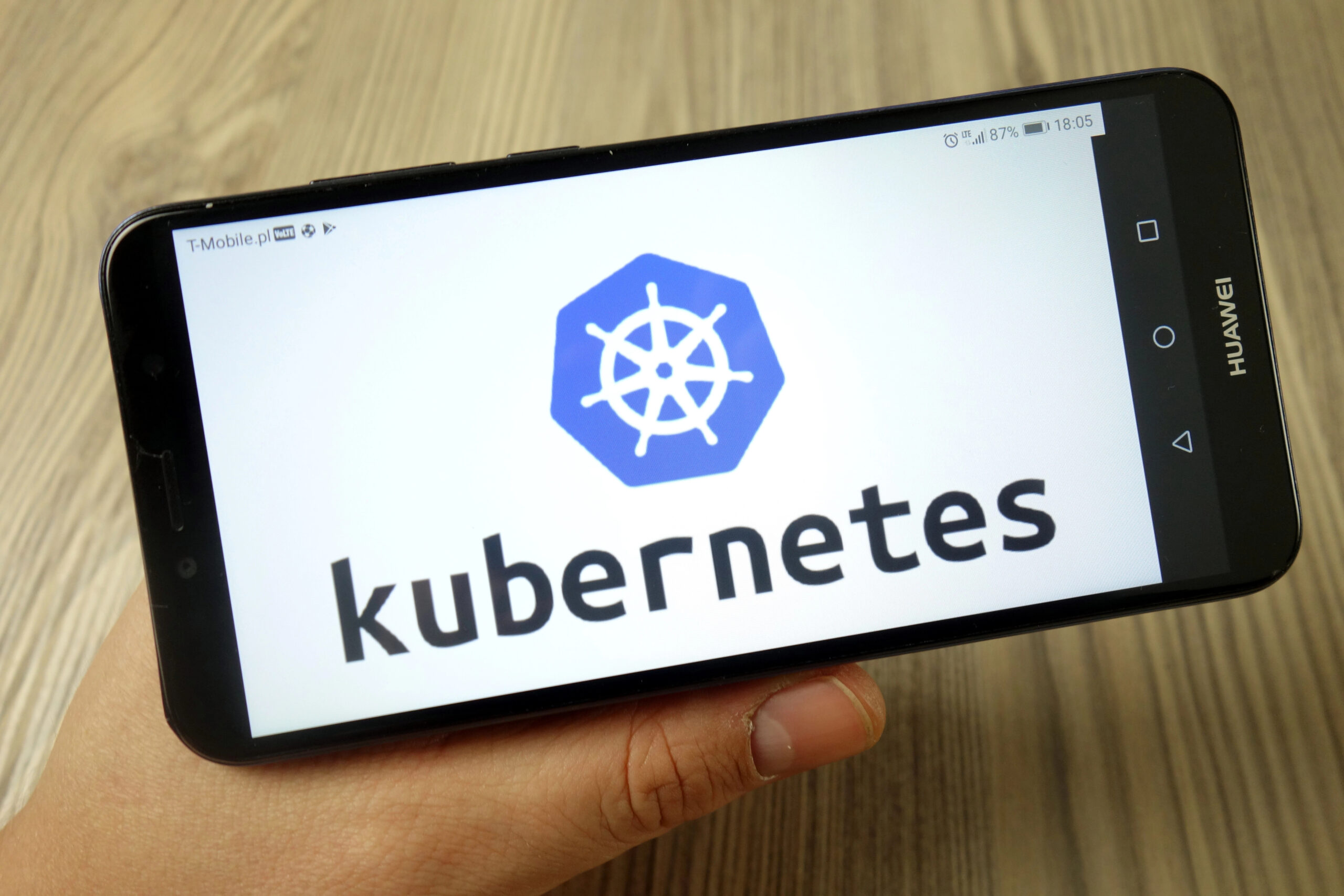
Gateway API Inference Extension
The collaboration between Google, ByteDance, and Red Hat has yielded significant improvements to Kubernetes’s capabilities for handling generative AI workloads. One of the key developments is the Gateway API Inference Extension, which revolutionizes the way model inference is managed at scale. This extension enables intelligent routing for Parameter-Efficient Fine-Tuning (PEFT) techniques, with a particular focus on Low-Rank Adaptation (LoRA). By optimizing the routing process, organizations can achieve higher efficiency and better resource utilization when deploying large language models.
Dynamic Model Management
To further enhance Kubernetes’ AI capabilities, new APIs have been introduced: InferencePool and InferenceModel. These APIs facilitate the dynamic loading of fine-tuned models, allowing for greater flexibility and adaptability in AI workloads. This advancement is particularly crucial for organizations that need to switch between different model versions or deploy specialized models on-demand, without incurring significant downtime or resource overhead.
Resource Allocation and Performance Insights
The collaboration has also resulted in the development of Dynamic Resource Allocation, a feature that automates the scheduling of GPUs and TPUs. When used in conjunction with the vLLM inference engine, this feature significantly enhances resource management efficiency. Additionally, a comprehensive benchmarking project has been launched to provide detailed performance insights on model servers and accelerators. These tools empower organizations to make data-driven decisions when scaling their AI applications, ensuring optimal performance and cost-effectiveness.
Enhancing Kubernetes AI Workloads with the Gateway API Inference Extension
The Gateway API Inference Extension represents a significant leap forward in managing AI workloads within Kubernetes environments. This innovative feature addresses the growing need for efficient handling of Parameter-Efficient Fine-Tuning (PEFT) techniques, particularly Low-Rank Adaptation (LoRA).
Streamlining Model Inference
By introducing intelligent routing capabilities, the Gateway API Inference Extension optimizes the flow of inference requests. This enhancement allows you to dynamically direct traffic to the most appropriate fine-tuned models, ensuring optimal performance and resource utilization. The extension’s sophisticated routing logic considers factors such as model specialization and current load, enabling more efficient processing of diverse inference tasks.
Enhancing Scalability and Flexibility
With the Gateway API Inference Extension, you can seamlessly scale your AI infrastructure to accommodate growing demands. It provides a flexible framework for managing multiple fine-tuned models, allowing you to easily deploy and switch between different versions as needed. This adaptability is crucial in scenarios where rapid iteration and deployment of AI models are essential.
Improved Resource Management
The extension’s intelligent routing capabilities contribute to better resource allocation across your Kubernetes cluster. By directing requests to the most suitable models, you can avoid bottlenecks and ensure that your computational resources are used efficiently. This optimization translates to improved overall performance and cost-effectiveness in your AI operations.
Extending Gateway API Inference Extension for Dynamic Model Loading with InferencePool and InferenceModel
Revolutionizing Model Management
The introduction of InferencePool and InferenceModel APIs marks a significant leap in Kubernetes’ ability to handle AI workloads efficiently. These new APIs address the critical need for the dynamic loading of fine-tuned models, a capability that’s becoming increasingly important as AI applications grow in complexity and scale.
InferencePool allows you to manage a collection of related models, facilitating seamless switching between different versions or variations. This API is particularly useful when you’re dealing with multiple fine-tuned models tailored for specific tasks or domains. By grouping these models together, you can optimize resource allocation and streamline model selection processes.
Enhancing Flexibility and Performance
The InferenceModel API complements InferencePool by providing granular control over individual model instances. With this API, you can dynamically load, unload, and update models without disrupting the entire application. This level of flexibility is crucial for maintaining high availability and performance in production environments.
These APIs work in tandem to enable:
Rapid model updates and A/B testing
Efficient resource utilization through dynamic scaling
Improved fault tolerance and system reliability
By leveraging these new APIs, you can create more responsive and adaptable AI systems that can quickly adjust to changing requirements or data patterns. This dynamic approach to model management represents a significant step forward in making Kubernetes an even more powerful platform for large-scale AI deployments.
Benchmarking AI Performance with Gateway API Inference Extension and Model Accelerators
Comprehensive Performance Analysis
The launch of a dedicated benchmarking project marks a significant step forward in understanding and optimizing AI workloads on Kubernetes. This initiative aims to provide detailed performance insights on various model servers and accelerators, enabling developers and operations teams to make informed decisions when scaling their AI applications.
By systematically evaluating different configurations, the project offers valuable data on throughput, latency, and resource utilization across a range of scenarios. This information is crucial for effectively managing resources and ensuring optimal performance in large-scale AI deployments.
Practical Applications and Benefits
The benchmarking results offer practical benefits for organizations leveraging Kubernetes for AI workloads:
Resource allocation optimization: Teams can fine-tune their infrastructure based on empirical data, maximizing efficiency and cost-effectiveness.
Performance prediction: Accurate benchmarks enable better capacity planning and help set realistic expectations for model inference times.
Accelerator selection: Comparisons between different GPU and TPU configurations assist in choosing the most suitable hardware for specific workloads.
Continuous Improvement and Adaptation
As AI technologies evolve rapidly, this benchmarking project serves as a living resource. Regular updates and expansions of the test suite ensure that the insights remain relevant and valuable. By incorporating feedback from the community and adapting to new hardware and software developments, the project helps Kubernetes maintain its position as a robust platform for cutting-edge AI applications.
Dynamic Resource Allocation: Automating GPU and TPU Scheduling for Efficient Inference
Revolutionizing Resource Management
Dynamic Resource Allocation (DRA) represents a significant leap forward in the management of GPU and TPU resources for AI workloads. Developed through a collaboration between Google, ByteDance, Red Hat, and Intel, this innovative feature automates the scheduling of these critical computational assets. By intelligently allocating and deallocating resources based on real-time demand, DRA optimizes the utilization of expensive hardware, ensuring that your AI inference tasks run with maximum efficiency.
Seamless Integration with vLLM
When paired with the vLLM inference engine, DRA truly shines. This powerful combination allows for dynamic scaling of resources, adapting to fluctuating workloads with minimal latency. As demand increases, additional GPUs or TPUs are automatically provisioned, ensuring that your models maintain peak performance even under heavy loads. Conversely, when demand subsides, excess resources are promptly released, optimizing cost-efficiency.
Enhancing Kubernetes for AI Workloads
The introduction of DRA positions Kubernetes as an even more robust platform for deploying large-scale AI applications. Automating resource management alleviates the burden on DevOps teams, reducing the need for manual intervention and fine-tuning. This not only streamlines operations but also allows for more predictable scaling of AI inference workloads, making it easier to plan and budget for infrastructure needs.
In Summary
As you’ve seen, the collaboration between Google, ByteDance, and Red Hat is pushing the boundaries of what’s possible with Kubernetes in the realm of AI. These advancements are not just incremental improvements; they represent a significant leap forward in how we deploy and manage large-scale AI applications. By addressing key challenges in model inference, resource allocation, and performance benchmarking, these innovations are set to redefine the landscape of AI infrastructure. As you consider your own AI initiatives, keep these developments in mind. They offer new possibilities for scaling your models efficiently and effectively, potentially unlocking new levels of performance and cost-effectiveness in your AI deployments.
More Stories
Alibaba’s $53 Billion AI and Cloud Investment: A Strategic Leap into the Future
As technology rapidly evolves, Alibaba’s $53 billion investment in AI and cloud computing deserves your full attention. This strategic move puts the Chinese e-commerce giant at the leading edge of a technological revolution.
PAL e-Wallet: Philippine Airlines’ Digital Leap into Seamless Travel Payments
Philippine Airlines (PAL) has taken a significant step forward with the launch of its PAL e-Wallet. This innovative digital platform represents a leap into the future of seamless travel payments, designed to enhance your journey from booking to boarding.
Avatars Ascend: YouTube’s Virtual Influencers Redefine Digital Fame
In the ever-evolving landscape of digital media, a new phenomenon is reshaping the concept of online celebrity. Virtual influencers, AI-generated or digitally animated personas, are rapidly ascending the ranks of YouTube stardom.
Reels Remix: Instagram’s Blend Turns DMs into Shared Discovery Zones
Have you ever wished you could explore Instagram Reels with your friends, discovering content that appeals to both of your interests?
ASUS AiCloud Bug Exposes Home Networks to Remote Attacks
Are you aware that your home network could be at risk? A recently discovered vulnerability in ASUS AiCloud-enabled routers has sent shockwaves through the cybersecurity community. This critical flaw, identified as CVE-2025-2492, exposes your network to potential remote attacks, allowing unauthorized access to your devices.
Samsung SmartThings Evolves: AI-Powered Automation and Ambient Sensing Redefine the Smart Home Experience
Samsung SmartThings redefines your connected living experience. With the introduction of AI-powered automation and ambient sensing capabilities, SmartThings is elevating home management to unprecedented levels of sophistication and convenience.