AMD’s latest foray into the world of artificial intelligence chips has been long awaited. They’ve been busy at work designing new silicon that will bring enhanced performance and energy efficiency to data centers across the globe. The innovative designs allow companies to effectively train massive neural networks while keeping costs down. There is much to be excited about with AMD’s new offerings that are optimized specifically for AI workloads. The specialized hardware provides a significant competitive edge that could shake up the industry. Stay tuned as we dive into the details and examine how AMD’s fresh designs will empower organizations to accomplish more with artificial intelligence.
AMD Unveils New AI Chip Lineup
Accelerated Computing for Data Centers AMD recently announced new AI chips designed to accelerate computing capabilities in data centers, focusing on higher performance and improved energy efficiency. The chips are based on AMD’s new Zen 4 CPU architecture and RDNA 3 GPU architecture, which provide enhanced performance for training and inference of AI models.
Zen 4 Architecture
- The Zen 4 architecture offers next-generation CPU cores with increased machine learning performance. It provides up to 35% higher performance for ML workloads versus the previous generation. The improved performance comes from larger caches, higher clock speeds, and an updated microarchitecture. For data centers, the Zen 4 architecture provides more throughput for ML tasks like natural language processing using technologies such as transformers.
RDNA 3 Architecture
- AMD’s RDNA 3 architecture includes a new GPU IP specialized for ML workloads. It offers up to 54% higher performance for ML training and up to 47% higher performance for ML inference versus AMD’s previous generation. The RDNA 3 architecture includes enhanced compute units optimized for ML, high-bandwidth memory controllers, and lossless compression engines to improve bandwidth and reduce latency. For data centers, the increased ML performance of RDNA 3 accelerates workloads such as computer vision, speech recognition, and recommendation systems.
Optimized Software Stack
- To fully utilize the new hardware capabilities, AMD provides an optimized open software stack for data center AI. This includes optimized versions of frameworks such as PyTorch, TensorFlow, and MXNet, as well as libraries like BLAS, cuDNN, and NCCL. AMD works closely with ML framework developers to ensure maximum utilization of AMD hardware. The open software stack provides data centers flexibility and helps avoid vendor lock-in.
Overall, AMD’s latest AI chips and software stack significantly accelerate ML workloads in data centers. With a focus on both training and inference, as well as CPU and GPU hardware, AMD provides data centers with a complete solution for developing and deploying AI models. The open standards-based approach helps maximize performance and flexibility.
Key Features of AMD’s Latest AI Chips
Enhanced Compute Capabilities
- AMD’s new AI chips provide significantly improved computing capabilities over previous generations. The chips offer up to 2.5 times higher peak FLOPS for AI training and up to 30% higher performance for AI inference workloads compared to AMD’s prior generation. This enhanced performance allows data scientists and researchers to build more accurate AI models in less time.
Optimized for Popular Frameworks
- The chips have been optimized to accelerate the performance of popular AI software frameworks like TensorFlow, PyTorch, and MXNet. The optimizations improve performance for key AI workloads like computer vision, natural language processing, and recommendation systems. Data scientists can leverage the optimized frameworks to improve the performance of their AI applications without significant code changes.
Improved Power Efficiency
- AMD’s latest AI chips deliver up to 50% better power efficiency than AMD’s previous generation. The improved power efficiency reduces infrastructure costs and environmental impact. It allows data centers to operate AI workloads at a lower cost and supports more sustainable AI progress.
Flexible and Scalable
- The new AI chips are designed to flexibly accelerate both training and inference workloads. They scale from entry-level single-socket servers up to high-performance multi-socket servers, providing a range of options for different performance and cost requirements. The scalability enables data scientists and researchers to start small and scale their AI infrastructure over time as needed.
In summary, AMD’s latest AI chips provide significant performance, efficiency, and scalability improvements for accelerating AI workloads. The enhanced capabilities allow data scientists and researchers to build more powerful AI models faster and at lower cost. AMD continues to push the boundaries of AI innovation with its optimized and flexible new chips.
How AMD’s New Chips Enhance AI Performance
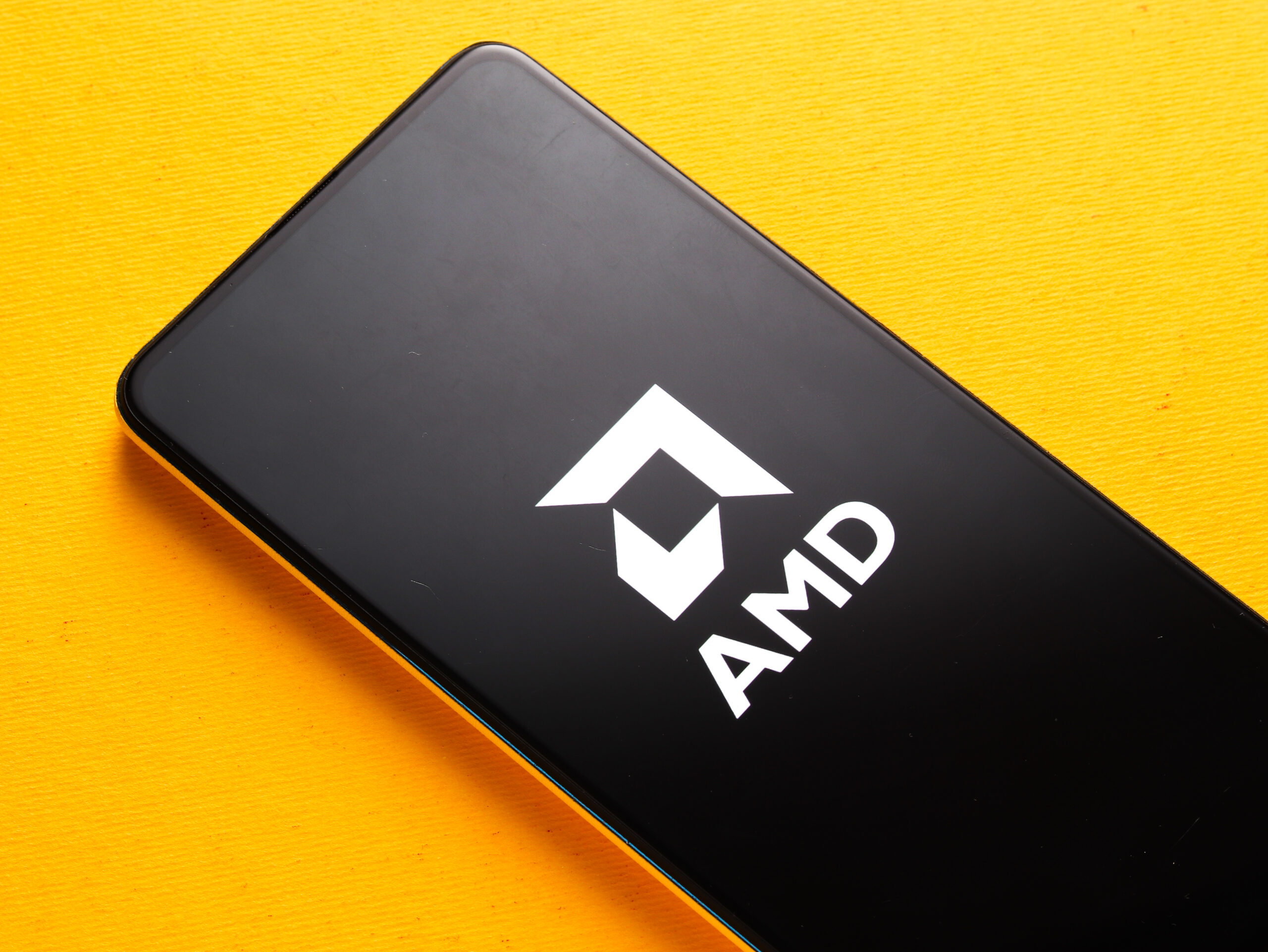
AMD’s latest central processing units (CPUs) and graphical processing units (GPUs) are designed to accelerate artificial intelligence (AI) workloads and enhance the performance of AI models. The chips provide improved deep learning performance due to architectural optimizations and support for mixed precision computing.
CPU Optimizations for AI
- AMD’s latest EPYC server CPUs feature dedicated AI accelerators to speed up deep learning training and inference. The chips support matrix multiplication instructions that can execute multiple multiply-and-add operations in a single clock cycle. This results in higher performance for AI models with large numbers of parameters. The CPUs also have large on-chip caches to keep AI model data close to the computing cores.
GPUs Purpose-Built for Deep Learning
- AMD’s new Radeon Instinct MI100 GPUs are built from the ground up for AI and high-performance computing. They provide ultra-fast floating point performance to accelerate deep learning training. The GPUs feature new compute units optimized for mixed precision computing, supporting FP64, FP32, and INT4/INT8 precisions in hardware. This allows AI models to use the precision that suits each layer best, maximizing performance.
Open Software Ecosystem for AI
- To simplify AI deployments, AMD’s new hardware works with popular open-source deep learning frameworks like PyTorch and TensorFlow. AMD also contributes to the open-source ROCm software platform, which provides optimizations for AI and HPC on AMD GPUs. Using open and optimized software helps data scientists and researchers get the most out of AMD hardware for AI.
With purpose-built hardware and software for artificial intelligence, AMD’s latest chips demonstrate the company’s commitment to driving AI innovation. By enhancing deep learning performance and efficiency, the new CPUs and GPUs aim to accelerate discoveries that can benefit both businesses and society. Overall, AMD has delivered on its promise of continued improvements in computing technologies to push the boundaries of AI.
Comparing AMD’s AI Chips to Competitors
AMD’s latest 7nm EPYCTM processors and AMD InstinctTM accelerators are designed specifically for AI and HPC workloads in data centers. These new chips provide higher performance and power efficiency than competitors.
Processing Power
- AMD’s 3rd Gen EPYC processors feature up to 64 cores and 128 threads, enabling significantly higher throughput for AI and ML workloads compared to competitors. The 7nm process technology also allows AMD to pack more transistors into each chip, which translates into higher performance within the same power envelope.
Memory Bandwidth
- AMD’s chips are connected with its Infinity Architecture, which provides up to 400GB/s of bandwidth versus 10-50GB/s in competitors’ offerings. The high bandwidth allows fast access to large amounts of data, enabling higher performance for memory-intensive AI training workloads.
Flexible Architecture
- AMD’s chips support a variety of interconnect standards like PCIe 4.0 and CCIX 1.0. The flexible architecture makes it easy to build systems optimized for different workloads. The chips also support key AI frameworks like TensorFlow, PyTorch, and MXNet.
Energy Efficiency
- AMD’s 7nm chips are extremely power efficient, consuming up to 50-90% less power than 14nm chips. The power savings can significantly reduce costs for AI data centers, especially when running at high utilization. AMD estimates that its latest EPYC chips could reduce the total cost of ownership for a data center by up to 50% over 3-5 years compared to older server CPUs.
In summary, AMD’s latest AI chips provide substantially higher performance, memory bandwidth, and power efficiency compared to competitors’ offerings. The flexible architecture and support for major AI frameworks also make AMD’s solutions ideal for building optimized systems for a wide range of AI workloads. For data center operators focused on performance, cost, and sustainability, AMD’s 7nm EPYC processors and Instinct accelerators are compelling options.
The Future of AMD’s AI Chip Development
AMD has accelerated its AI chip roadmap to stay ahead of the competition. Faster, More Efficient Chips AMD will continue improving its AI chips with each new generation, focusing on higher performance and greater energy efficiency. The company aims to develop faster chips that can handle increasingly complex AI and machine learning workloads. At the same time, AMD will optimize its chips to minimize power consumption since many data centers prioritize sustainability and lower operating costs.
Broader Range of AI Chips
- AMD currently offers AI chips for training and inference, but will likely expand into other segments. For example, AMD may release chips specifically tailored for edge computing or autonomous vehicles. The company could also develop chips for emerging AI applications like robotic process automation or conversational AI. By expanding into more AI segments, AMD can diversify its product portfolio and open up new revenue streams.
Partnerships and Acquisitions
- AMD may pursue strategic partnerships and acquisitions to bolster its AI chip capabilities. The company could collaborate with startups to develop innovative AI hardware or software technologies. AMD could also acquire smaller companies, especially those with valuable intellectual property or engineering talent. Partnerships and acquisitions are an effective way for AMD to gain new technical and domain expertise that would otherwise take years to build internally.
Competition from Larger Rivals
- While AMD has recently gained ground in the AI chip market, larger competitors like Intel, NVIDIA, and Google still pose a major threat. These companies have greater resources, technical capabilities, and influence in the AI space. To effectively compete, AMD must continue out-innovating rivals by releasing superior products on aggressive timelines. AMD also needs to strengthen its marketing and build tighter relationships with key customers and partners. With strong execution, AMD can solidify its position as a leader in high-performance, energy-efficient AI chips.
In summary, AMD is well-positioned to shape the future of AI chips but still faces significant challenges from much larger competitors. By accelerating innovation, diversifying its portfolio, and making strategic power moves, AMD can fulfill its goal of providing the AI chips that power breakthrough technologies. The AI chip market is still relatively nascent, leaving ample room for AMD to stake its claim.
Key Takeaways
The recent developments by AMD in AI chip technologies demonstrate their commitment to driving innovation and accelerating performance for high-demand workloads. With enhanced capabilities in areas like machine learning and natural language processing, these new offerings provide data centers and enterprises with powerful tools to derive insights, automate processes, and scale intelligently. While the long-term impacts remain to be seen, the potential is clearly there for AMD’s revamped portfolio to disrupt the AI silicon space in a big way. By focusing intently on next-gen architectures optimized for AI, AMD aims to carve out an even greater foothold in this burgeoning market. For any organization exploring how to most effectively harness artificial intelligence, AMD’s latest chips warrant a close look. The future is bright for those leveraging AI, and AMD is positioning itself at the forefront to power those efforts.
More Stories
Confluent Unifies Real-Time and Historical Data to Power Next-Gen AI and Analytics
Confluent has made major progress by upgrading its Confluent Cloud platform. These enhancements aim to unify data streams for advanced AI applications.
AI Synergy: Exabeam and Vectra AI Forge Unified Front Against Evolving Cyber Threats
The alliance between Exabeam and Vectra AI marks a major cybersecurity advancement. This strategic partnership merges top-tier technologies from both companies to enhance defenses against complex cyberattacks.
ChatGPT Deep Research Now Integrates with Dropbox and Box for Enhanced Data Access
ChatGPT Deep Research integration with Dropbox and Box enhances data access for users enabling them to link cloud storage accounts directly to Deep Research.
Next-Gen Xeon 6 CPUs Elevate Data Center AI Workloads in Nvidia DGX B300
In the rapidly evolving landscape of artificial intelligence, staying ahead requires cutting-edge technology that can efficiently handle complex workloads. You...
Apple Trims Features to Fast-Track Smart Home Hub Amid Siri AI Delays
At the core of these delays is the sluggish advancement in Siri AI enhancements, pivotal to the hub’s operation.
Apex Group Taps Tom Bennett to Spearhead Global Fintech Strategy
Apex Group Ltd. has strategically appointed Tom Bennett as Global Head of Fintech. This pivotal role highlights Apex Group’s commitment to advancing its digital capabilities across private markets, capital markets, and digital assets.