Business leaders understand the importance of protecting an organization’s data and assets. With cyber threats on the rise, security has become a top concern. Google aims to support these efforts by leveraging AI to enhance fraud detection across its cloud services. In this article, learn how Google is integrating sophisticated machine learning into its platforms, with advanced tools to combat fraudulent activity targeting systems and sensitive information. Discover how these AI capabilities allow Google to identify emerging fraud patterns and even predict future attacks before they occur. Stay ahead of cybercriminals by understanding Google’s latest innovations in AI-powered fraud defense. With knowledge of these new protections at your disposal, confidently build your digital initiatives on Google Cloud. Rest assured that the most valuable data is guarded by intelligent, automated security.
Overview of Google’s AI-Based Fraud Detection
Detecting Fraudulent Transactions
- Google uses machine learning models trained on historical transaction data to detect fraud in real time. The models analyze new transactions and score them based on the probability of fraud. If a transaction is flagged as fraudulent, Google notifies the enterprise promptly.
Anomaly Detection
- Google’s AI models establish a “normal” baseline of behavior for each user based on their transaction history and other signals. New transactions that deviate significantly from a user’s normal pattern are flagged as anomalies and reviewed for potential fraud. For example, if a user who normally makes small purchases suddenly makes an unusually large order, that transaction would be flagged as an anomaly.
Risk Scoring
- Google assigns a risk score to each transaction which represents the probability of fraud. Therefore, these risk scores is calculated based on factors like transaction amount, location, time, and device. Transactions with a high risk score are prioritized for review by Google’s fraud analysts. The risk-scoring system improves over time as Google’s AI models get more data to learn from.
Continuous Monitoring
- Google’s fraud detection systems monitor transactions continuously and can detect fraud even after a transaction has been completed. If suspicious activity is detected for a past transaction, Google will notify the enterprise immediately. Continuous monitoring allows Google to detect emerging fraud trends as they happen.
Google’s AI-based fraud detection offers robust, real-time security for enterprises using Google’s cloud platforms. By leveraging large datasets and advanced machine learning, Google is able to detect even the most sophisticated fraud attacks. With continuous monitoring and improvement, Google’s fraud detection systems provide enterprises with comprehensive protection for their business.
How Google Is Using AI to Detect Fraud
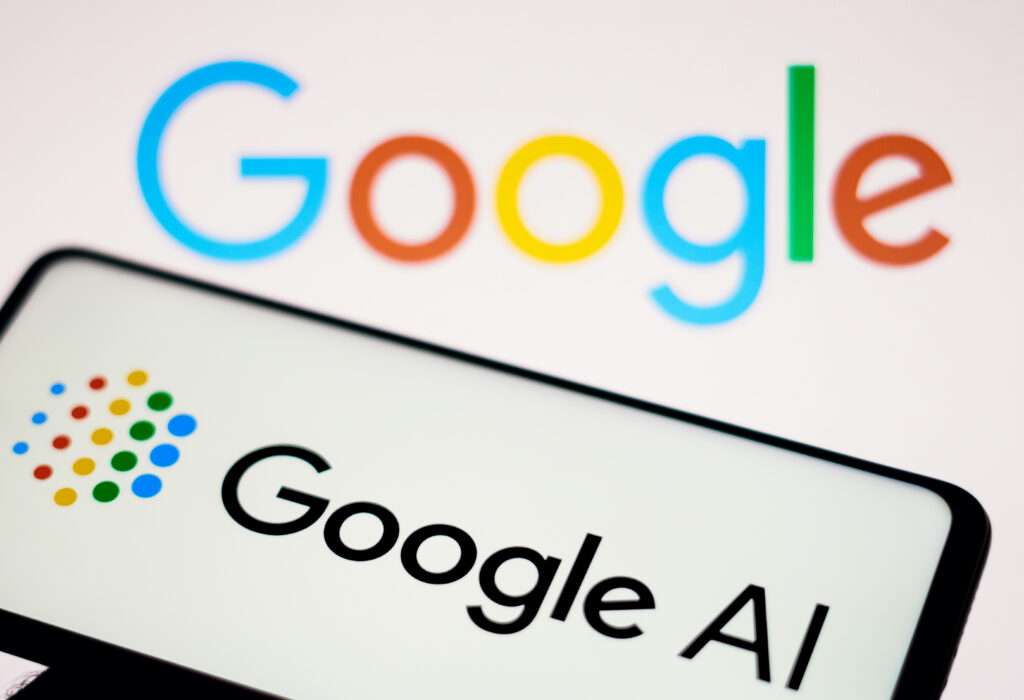
Google is deploying artificial intelligence models within its cloud services to identify and prevent fraudulent activity. Their AI-based fraud detection offers enhanced security for enterprises using Google platforms.
Detecting Anomalous Behavior
- Google’s AI models analyze user behavior and activity patterns to detect anomalies that could signal fraud. The models establish a baseline of normal behavior for each account and can then identify sudden changes that fall outside expected patterns. For example, if a user who normally accesses an account from New York suddenly logs in from Ukraine, the AI may flag that as potentially fraudulent.
Analyzing Large Data Sets
- Google’s AI systems can quickly analyze huge amounts of data to identify connections that humans might miss. Furthermore, AI looks at factors like location, time of access, IP address, and more to detect coordinated fraudulent efforts across accounts. For example, the AI may notice that several accounts accessed from the same IP address at the same time are exhibiting anomalous behavior, signaling an automated bot or hacking attempt.
Adapting to New Threats
- As fraudsters develop new techniques, Google’s AI models learn and adapt to detect emerging threats. The models are constantly trained on new data to expand their knowledge and identify the latest fraud patterns. The AI systems can then apply what they’ve learned to detect and block new fraudulent activity, staying one step ahead of malicious actors.
Providing Actionable Insights
- Google’s AI doesn’t just detect potential fraud, it provides detailed insights to help enterprises take appropriate action. The AI can pinpoint specific accounts or activities that are likely fraudulent, along with an explanation of why it has flagged them. Security teams can then quickly investigate further and take measures to prevent losses, block accounts, or patch vulnerabilities.
With AI and machine learning, Google is strengthening its fraud detection and security capabilities. Their technology helps safeguard enterprises and their users from the growing threat of online fraud. By partnering with Google, businesses can leverage these advanced AI systems to gain valuable intelligence and protect their platforms.
Benefits of AI-Based Fraud Detection for Enterprises
AI-powered fraud detection provides significant advantages for companies using Google’s cloud services. By leveraging machine learning models trained on massive datasets, Google can identify malicious activities with a high degree of accuracy.
Enhanced Security
- AI systems excel at detecting anomalies and subtle patterns that indicate fraudulent behavior. Google’s AI also monitors user accounts and APIs for signs of compromise, unauthorized access, and other threats. It can spot hackers, scammers, and malicious bots targeting enterprises much faster than human analysts alone. This additional layer of security protects sensitive customer data, financial information, and other digital assets.
Reduced False Positives
- Machine learning models become more accurate over time as they process more data. Google’s AI has analyzed billions of data points from its global network, giving it a significant advantage over rules-based systems. The AI can determine normal usage patterns and distinguish legitimate user behavior from fraudulent activity with a high degree of precision. This helps minimize false positives that can frustrate customers and damage the user experience.
Proactive Monitoring
- Unlike reactive anti-fraud solutions, Google’s AI proactively monitors for threats 24 hours a day, 7 days a week. The machine learning models look for anomalies in real time and send alerts as soon as potentially fraudulent behavior is detected. This constant vigilance and fast response time help mitigate risks before damage occurs. Issues can be addressed promptly, accounts can be secured, and preventative actions can be taken.
Improved Efficiency
- AI-based fraud prevention automates many of the tasks typically performed by human analysts. Google’s machine learning models can also handle the bulk of the monitoring, detection, and risk analysis. This allows security teams to focus their time and efforts on investigating and remediating the most serious threats. Staff can also spend more time on strategic initiatives to strengthen defenses rather than repetitive manual reviews. The end result is a more optimized anti-fraud program.
In summary, Google’s AI-powered fraud detection provides enterprises with advanced security, fewer false positives, continuous monitoring, and improved efficiency. By leveraging Google’s machine learning expertise and massive datasets, companies can better protect their digital assets from fraud.
Challenges and Limitations of AI for Fraud Detection
While AI has enabled Google to improve fraud detection in its cloud services, the technology also presents challenges and limitations. As AI models rely on large amounts of data to learn how to detect anomalies, they require massive datasets that are clean, unbiased, and representative in order to function effectively.
- Data Quality and Quantity
- AI models are only as good as the data used to train them. If there are gaps or biases in the training data, the models may fail to detect some types of fraud or generate false positives. Google must ensure it has enough high-quality data that encompasses the diversity of normal user behavior and known fraud patterns.
- Explainability
- Many AI techniques are based on complex algorithms and neural networks that are opaque and difficult for humans to understand. This lack of explainability makes it hard to determine why an AI model predicted fraud or to correct errors. Google’s models should provide explanations for their predictions to build trust in the systems and enable improvements.
- Adversarial Attacks
- As AI becomes more widely used for fraud detection, criminals are also developing new techniques to evade AI systems. Adversarial examples are inputs designed to fool machine learning models by exploiting their vulnerabilities. Google must safeguard against adversarial attacks by building robust, resilient AI models and updating them frequently as new threats emerge.
- Bias
- If the data used to train AI models reflects biases, the models may discriminate unfairly against some groups. Google must ensure its AI systems avoid bias by relying on diverse, inclusive data and by subjecting models to rigorous testing. Detect any biases by retraining the models.
AI has significant potential for improving fraud detection, but it also brings risks and challenges that Google must consider seriously. With continual progress in explainability, data quality, model robustness, and inclusiveness, Google can maximize the benefits of AI for fraud detection while minimizing its limitations. It is important to practice constant vigilance and commit to responsible development as AI becomes more advanced and the deployment range grows wider in the future.
The Future of AI in Fraud Detection and Cybersecurity
Google leads the way in deploying AI models to detect and prevent fraud. Their cutting-edge technology is set to transform fraud detection and cybersecurity.
Real-Time Monitoring
- AI models can monitor transactions and user behavior in real-time to identify anomalies that could indicate fraud. Machine learning algorithms detect subtle patterns that humans may miss, enabling Google to flag suspicious activity immediately. Real-time monitoring improves fraud detection and allows security teams to respond faster.
Personalized Security
- AI enables highly personalized security solutions based on each user’s activity and risk level. Google also tailor security settings for maximum protection of customers’ accounts and data. AI models also adapt security strategies as new fraud and cyber threats emerge. Personalized security powered by AI helps ensure the optimal balance of protection and convenience for all Google Cloud users.
Scalable and Cost-Effective
- AI-based fraud detection is highly scalable and cost-effective. Google’s machine learning models can monitor massive amounts of data across billions of users and devices. AI requires fewer human resources to implement and maintain, reducing operating costs. The scalability and efficiency of Google’s AI and machine learning technology enable affordable advanced security solutions for organizations of all sizes.
Continuous Improvement
- Google’s AI models leverage machine learning to continuously improve fraud detection over time. As the models analyze more data, their accuracy and sophistication increase. Google also uses human experts to evaluate the AI’s performance and make adjustments to algorithms. The combination of machine learning and human oversight allows the AI to adapt to new fraud techniques and strengthen security across Google’s services. Continuous improvement will be crucial as cybercriminals become more adept at leveraging AI for malicious purposes.
With AI and machine learning at the forefront of their strategy, Google is transforming how enterprises prevent and mitigate fraud. Advanced capabilities like real-time monitoring, personalized security, scalability, and continuous improvement will shape the future of fraud detection and cybersecurity. By deploying Google’s AI technology, organizations can gain a robust defense against emerging threats.
To Conclude
You have seen how Google utilizes AI for enhanced fraud detection in its cloud services. Implementing deep learning models allows Google to analyze vast datasets, identifying patterns and anomalies indicative of fraudulent activity. This provides a major security advantage to companies hosting data and applications in Google Cloud. While AI is not impervious, the speed and scalability it offers establish a strong frontline defense. Moving forward, expect to see Google continue expanding its AI fraud prevention as part of a comprehensive cybersecurity strategy. With powerful technology and an ongoing commitment to enterprise security, Google Cloud emerges as an attractive option for your business.
More Stories
Meta AI App Adds Warning to Prevent Accidental Sharing of Private Chats
In today's world, digital privacy is more important than ever. Meta has taken a major step to protect user data....
Android Instant Apps Retired After Years of Low Adoption
Google decides to retire Android Instant Apps by December 2025 as they struggled to capture user interest since its launch in 2017.
OpenAI Cracks Down on State Sponsored Abuse of ChatGPT
OpenAI has taken a decisive step to thwart state-sponsored exploitation of its ChatGPT technology. As detailed in a comprehensive threat intelligence report, OpenAI has disabled numerous accounts implicated in cyber operations orchestrated by state actors from countries including Russia, China, and North Korea.
Record‑Breaking Rupiah Loan Powers AI‑Ready Batam Data Hub
They secured a historic record-breaking Rupiah 6.7 trillion loan from financial giants DBS and UOB. This monumental financing supports an AI-ready data center campus at Batam’s Nongsa Digital Park.
Google Closes Loophole That Let Hackers Bruteforce Your Hidden Phone Number
Recently, Google took decisive action to close a significant vulnerability that could potentially expose users’ hidden phone numbers to malicious actors.
Instagram Edits Brings In-App Teleprompter to Boost Creator Confidence
Introducing the in-app teleprompter within Instagram Edits, a tool designed to bolster your on-camera confidence and enhance viewer engagement.