Now, an advanced degree or extensive coding skills is not necessary to build intelligent applications anymore. The democratization of artificial intelligence is here. Smaller, more efficient language models that require less computational power are opening up the world of advanced natural language processing. These streamlined models enable faster deployment and scalability whilst maintaining high levels of accuracy and versatility. Now developers worldwide can integrate powerful AI into their products and services. The playing field is leveling. With the right tools, anyone can capitalize on transformative technologies like chatbots, semantic search, and sentiment analysis. Small Language Models are the key that unlocks AI capabilities for all.
What Are Small Language Models (SLMs)?
Small Language Models (SLMs) are compact neural networks designed to understand and generate human language. Unlike traditional large language models that require enormous datasets and computing power to train, SLMs can achieve high performance with far fewer parameters and less data. SLMs utilize efficient model architectures and pre-training techniques to capture semantic knowledge from relatively small datasets.
For example, a small language model may have 10 million parameters compared to the 175 billion parameters of a large model like GPT-3. Despite their reduced size, SLMs can reach 99% of the performance of much larger models on many NLP tasks.
The compact nature of SLMs enables faster training, lower latency, and greater scalability. Developers can deploy SLMs in environments where larger models are impractical due to memory or computational constraints. SLMs also facilitate privacy-preserving technologies by operating on sensitive data locally without relying on massive datasets. While large language models have revolutionized fields like conversational AI, their immense resource requirements remain prohibitive for most organizations and individual developers.
SLMs help democratize advanced NLP capabilities by providing an accessible and scalable alternative to unwielding large language models. With continued progress in model efficiency and data optimization techniques, small language models may ultimately surpass today’s largest models. SLMs represent an exciting new frontier that expands the possibilities of AI for organizations of all sizes. By optimizing models for performance, privacy, and practicality, small language models can help unlock the potential of artificial intelligence.
Benefits of Smaller Model Size
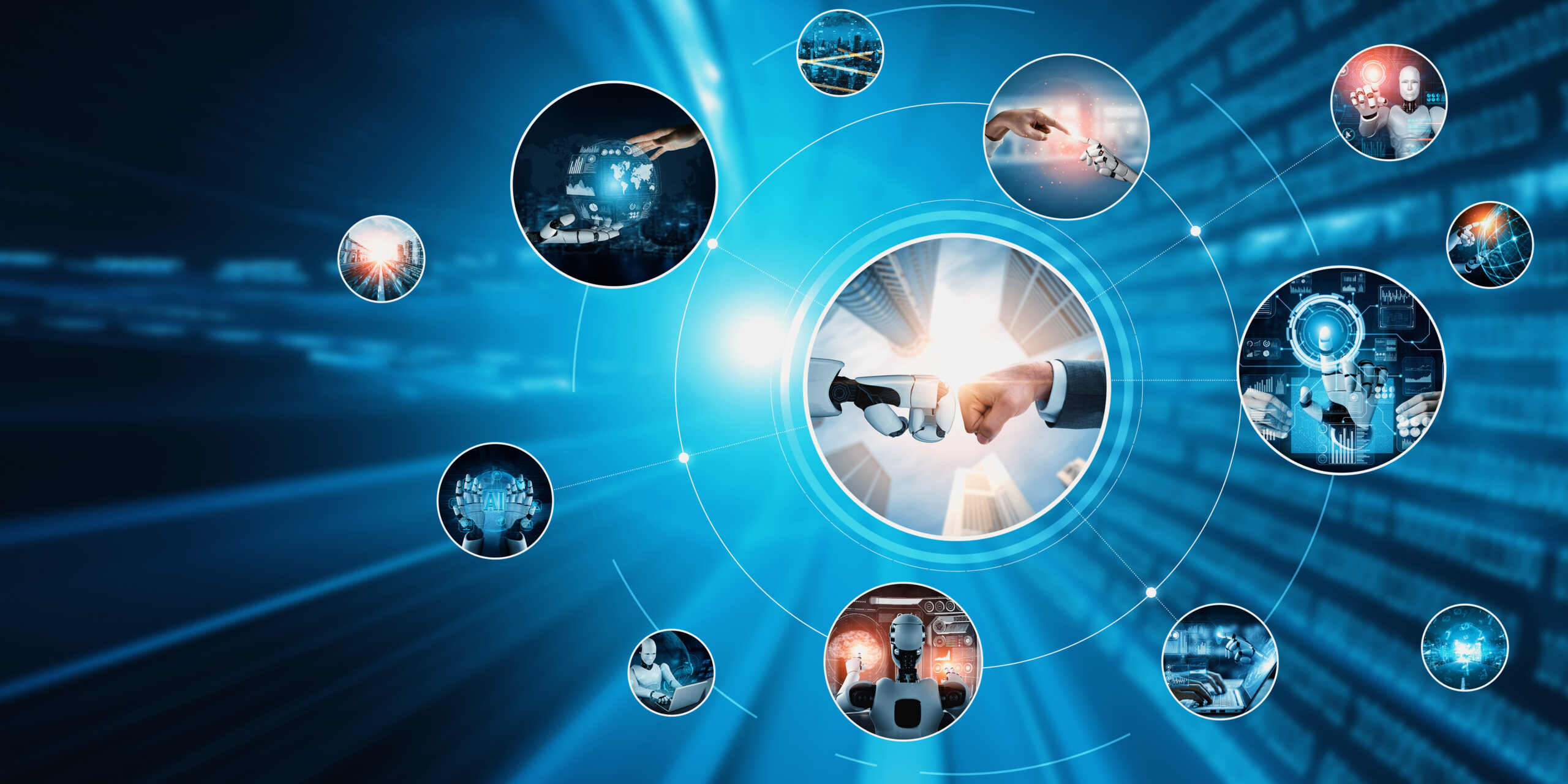
Faster Training and Deployment
- Smaller models require less data and computing power to train, enabling faster development and deployment cycles. For example, a model with hundreds of millions of parameters can take weeks to train on specialized hardware, whereas a model with tens of millions of parameters may train in hours or days on a single GPU. Faster training allows researchers and engineers to iterate more quickly and ship production models sooner.
Scalability
- Smaller models are more scalable since they are less computationally intensive. Multiple models can run simultaneously on the same hardware, and models can be deployed on less powerful devices like mobile phones or embedded systems. Democratizing AI by bringing its capabilities to more platforms and users.
Maintained Accuracy and Versatility
- While large models have achieved state-of-the-art results on some tasks, smaller models can still reach high accuracy on many NLP problems. For instance, models with hundreds of millions of parameters only slightly outperform models with tens of millions of parameters on sentiment analysis and question answering. Smaller models are also capable of handling a diverse range of NLP tasks, from classification and regression to summarization and translation.
Privacy Preservation
- Smaller models have fewer parameters, so they capture less personal information from training data. This can help address privacy concerns with neural networks. Using a smaller model or differentially private training are two options for building AI systems that leverage personal data while upholding individuals’ privacy.
In summary, smaller and more efficient models make advanced AI more accessible. They have significant benefits with manageable trade-offs in accuracy and should continue enabling progress in the democratization of artificial intelligence.
SLMs Increase Accessibility of AI
As AI has advanced, many of its capabilities have required extensive computational resources and highly specialized expertise to develop and deploy. Smaller language models (SLMs) are changing this by making AI more accessible to a wider range of developers and users.
Lower Compute Requirements
- SLMs can function effectively with a fraction of the data and computing power of large language models. For example, a model with hundreds of millions of parameters may only require a single GPU to train, rather than racks of high-end servers. This reduced cost and infrastructure enables more developers and organizations to experiment with and apply AI.
Faster Experimentation
- The decreased data and computing needs of SLM translate to faster development cycles. Changes to the model architecture or training data can be tested in hours or days instead of weeks. This speed allows for more rapid experimentation, enabling developers to try new techniques and optimize models more efficiently.
Scalability
- In addition to faster initial development, the lightweight nature of SLMs makes them easier to deploy at scale. The models can be exported to run locally on user devices or scaled to serve large numbers of users in production environments. This flexibility and scalability further expand the potential use cases and audiences that can benefit from AI.
Domain Adaptability
- While large language models require massive datasets to train on broad domains, SLMs can achieve strong performance with less data by focusing on specific domains. The models can be optimized for areas like healthcare, finance, or education by using datasets and pre-training techniques targeted to the domain. This adaptability provides more relevant and useful AI applications for various industries and subject areas.
In summary, smaller language models are democratizing AI by making its capabilities more accessible and applicable to a wider range of developers, organizations, and end users. With their reduced requirements and increased flexibility, SLMs are poised to enable the next wave of AI innovation.
Democratization of AI With SLMs
Less Complex Models, Broader Access
- Smaller language models are more computationally efficient, require less data to train, and are faster to deploy. This makes advanced AI capabilities accessible to a wider range of developers and organizations. Instead of needing specialized hardware and significant funding to build and train a large model, developers can now implement SLMs using resources they already have.
Quicker Iteration and Experimentation
- The reduced complexity of SLM also means that developers can iterate and experiment more quickly. They can retrain models on new data or try different architectures in hours or days instead of weeks or months. This accelerated experimentation enables developers to gain insights faster and build more sophisticated applications.
Customization for Specific Use Cases
- While large models aim to handle a broad range of natural language tasks, SLMs can be tailored for particular use cases. Developers can choose model sizes and architectures suited for their needs, and train the models on domain-specific datasets. These customized SLMs may achieve higher performance on specialized applications than a one-size-fits-all large model.
Opportunities for Under-Resourced Languages
- SLMs lower the barriers to developing AI systems in languages with fewer data resources. It is difficult to train a high-performing large model without huge datasets, but smaller models can achieve good results with less data. This makes it more feasible for developers to build AI for under-resourced languages that lack large datasets. SLMs could help address the imbalance in AI progress across languages.
In summary, smaller, more efficient language models are making advanced natural language processing capabilities more accessible. SLMs provide opportunities to accelerate experimentation, tailor models for specific use cases, and make progress in under-resourced languages. By lowering the barriers to AI development, SLMs have the potential to democratize artificial intelligence.
The Future of AI Democratisation
Increased Accessibility
- As SLMs become more advanced and efficient, AI will become more accessible to a wider range of developers. SLMs require less data and computing power, enabling faster deployment and scalability while maintaining high accuracy and versatility. This makes AI more affordable and practical for small companies and individual developers to implement.
Customised Solutions
- With greater access to AI, developers will be able to build customized solutions tailored to their specific needs. They can choose from a range of pre-trained models and fine-tune them with their own data. As models become more specialized, they will achieve higher performance on the target tasks. Specialized AI has the potential to greatly improve processes and experiences in many industries.
Decentralized Development
- The democratization of AI will lead to more decentralized development. Rather than a few large tech companies dominating the field, many independent developers and startups will drive progress. This could accelerate the rate of AI innovation as more people contribute their skills, ideas, and data. However, it also introduces risks around data privacy, security, and bias that will need to be addressed. Regulation and guidelines may be required to ensure AI development is done responsibly.
Broader AI Applications
- With AI more widely available, it will be applied to an increasing range of tasks and domains. As models become more versatile, they can be transferred between different applications, enabling new use cases. Democratized AI has the potential to positively impact society in fields like healthcare, education, transportation, and more. However, its effects will depend on how responsibly and ethically it is developed and applied.
Overall, the democratization of AI through SLMs will make advanced capabilities more accessible and affordable. This will enable customized, decentralized development of AI and its application to a broader range of domains. However, appropriate safeguards and oversight will be needed to ensure responsible and ethical progress. With prudent management, democratized AI can be developed and applied in a way that benefits both individuals and society as a whole.
To Sum It Up
While large language models have captured attention, smaller, more efficient models actually enable broader access to AI. Their reasonable computational requirements and accuracy make advanced natural language processing viable for more developers. As SLMs become prevalent, expect to see enhancements across applications and industries. Democratization of AI through SLMs can fuel innovation and progress. The future looks bright as more minds engage with AI through these accessible new tools. Responsible development and use remain imperative, but SLMs help tear down the barriers restricting AI to an elite few.
More Stories
Microsoft Unveils Windows 365 Link : A Compact Gateway to Cloud Computing
Microsoft’s latest innovation, the Windows 365 Link, emerges as a game-changing solution for your business. This compact, fanless mini PC represents a significant leap forward in cloud connectivity, offering you a streamlined gateway to Windows 365 Cloud PCs
DOJ Targets Google’s Dominance: Potential Chrome Sale and Android Unbundling on the Horizon
The tech giant argues these measures are too extreme. However, the DOJ sees them as necessary to ensure a fair playing field. The outcome of this antitrust battle redefines interactions with search engines, browsers, and mobile devices for years to come.
Australia Moves to Set 16 as the Minimum Age for Social Media
As you navigate the ever-evolving landscape of digital communication, a significant shift is on the horizon in Australia. The nation's...
Interlock Ransomware Surge Threatens U.S. Healthcare Systems Amid Growing Cyber Vulnerabilities
As you navigate the complex landscape of healthcare cybersecurity, a new threat looms on the horizon. The Interlock ransomware group...
Madison Technologies’ AI-Powered Rail Safety Innovation Earns Top Global Cisco Award
As you navigate the complex landscape of rail safety innovation, you'll find that Madison Technologies has emerged as a true...
Why Google Cloud’s Mandatory MFA is a Game-Changer for Secure Access
In an era where cyber threats are evolving at an alarming rate, Google Cloud is taking a bold step to...